- 09/10/2023
- Category: Ethics of Technology
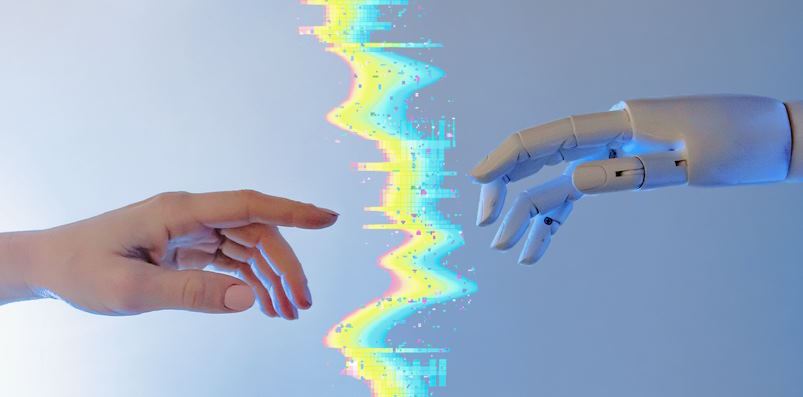
Ethical considerations play a central role in the responsible deployment of predictive analytics and ML in healthcare. By prioritising data privacy, mitigating bias, respecting patient autonomy, promoting transparency, and emphasising clinical utility, healthcare organisations can harness the potential of these technologies to enhance patient care while unveiling the ethical dilemmas of machine learning in healthcare and safeguarding patient welfare.
Data Privacy and Security
Predictive analytics and ML algorithms rely heavily on large datasets, including electronic health records (EHRs), medical imaging scans, and genetic information. Ensuring the privacy and security of sensitive patient data is paramount to maintaining trust and upholding ethical standards. Healthcare organisations must implement robust data protection measures, such as encryption, access controls, and anonymization techniques, to safeguard patient privacy and prevent unauthorised access or data breaches.
Bias and Fairness
Bias in predictive analytics and ML algorithms can perpetuate disparities in healthcare delivery and outcomes. Biassed algorithms may reflect historical inequalities in promoting transparency and accountability in healthcare AI or inadvertently discriminate against patient populations based on demographic factors, such as race, ethnicity, or socioeconomic status. Addressing bias requires transparent algorithmic development processes, diverse and representative training data, and ongoing monitoring and mitigation efforts to ensure fairness and equity in predictive models.
Informed Consent and Autonomy
Predictive analytics and ML in healthcare raise questions about patient autonomy and informed consent. Patients may be unaware of how their data is understood and the implications of predictive algorithms on their care. Respecting patient autonomy entails obtaining informed consent for data collection, analysis, and utilisation in predictive models.
Transparency and Explainability
Enhancing transparency and explainability requires developers to employ interpretable ML techniques, provide clear documentation of algorithmic processes, and facilitate open dialogue between stakeholders to foster understanding and trust in predictive analytics.
Clinical Utility and Benefit-Harm Balance
Predictive analytics and ML algorithms must demonstrate clinical utility and effectiveness to justify their integration into healthcare practice. While these technologies help to improve diagnostic accuracy, treatment selection, and ethical challenges in harnessing machine learning for patient care, their implementation. Additionally, healthcare providers must carefully weigh the benefits of predictive analytics against potential harms, such as overdiagnosis, overtreatment, and medicalization, to ensure the use of these technologies.